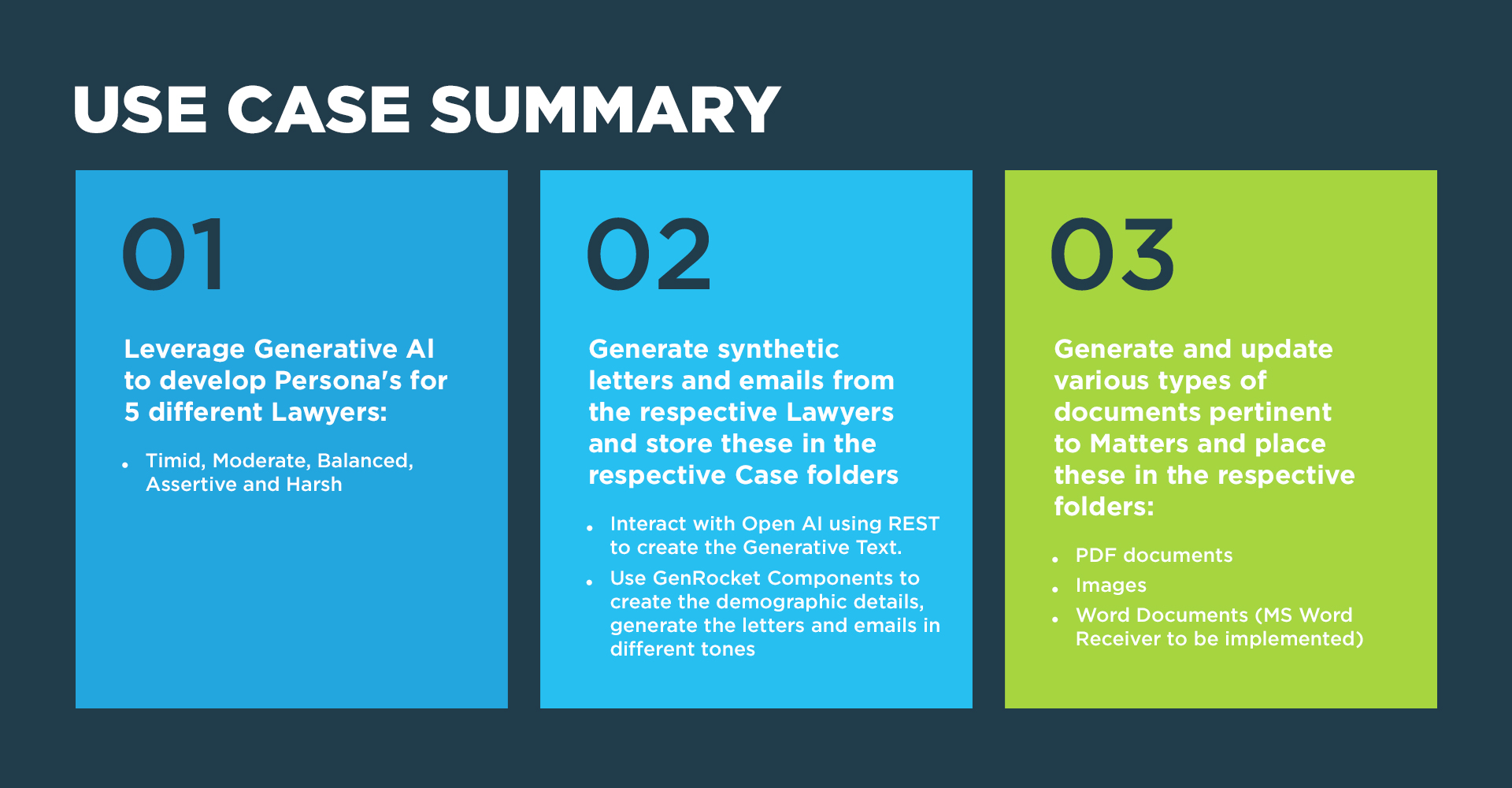
Synthetic Data Generation of Complex Documents with GenAI
Part 3: How a Prominent Legal Firm is Deploying the Technology
In this third part of our series on leveraging GenAI for controlled and accurate synthetic data generation, we delve into a real-world application of GenRocket’s platform, integrating the principles discussed in the previous two parts of the series. We will explore how GenRocket, combined with generative AI (GenAI), can address complex data provisioning needs, delivering robust synthetic data solutions at an enterprise scale by presenting a comprehensive use case deployed by a prominent legal firm.
Recapping Parts 1 and 2
In Part 1, we highlighted how GenAI can be leveraged by GenRocket’s Test Data Automation platform to generate high-quality synthetic data, ensuring data integrity and compliance while mitigating the risks associated with using GenAI tools by themselves. This approach allows organizations to create realistic and varied data sets that combine the use of structured and unstructured synthetic data without compromising sensitive information.
Part 2 focused on how the use of GenRocket’s platform can provide GenAI tools with enterprise scalability, showcasing its ability to manage the full data provisioning lifecycle and support enterprise-wide requirements for security, self-service, management and reporting. GenRocket’s scalable architecture ensures that businesses can handle large volumes of data efficiently, meeting the demands of modern data-driven environments.
Use Case Overview
A leading legal firm faced challenges in generating diverse and realistic data sets for training machine learning models used in the development of AI-assisted application software. To address these challenges, they turned to GenRocket’s platform, integrating it with GenAI to create synthetic data for various legal personas to generate legal correspondences, court pleadings, and other types of legal documentation.
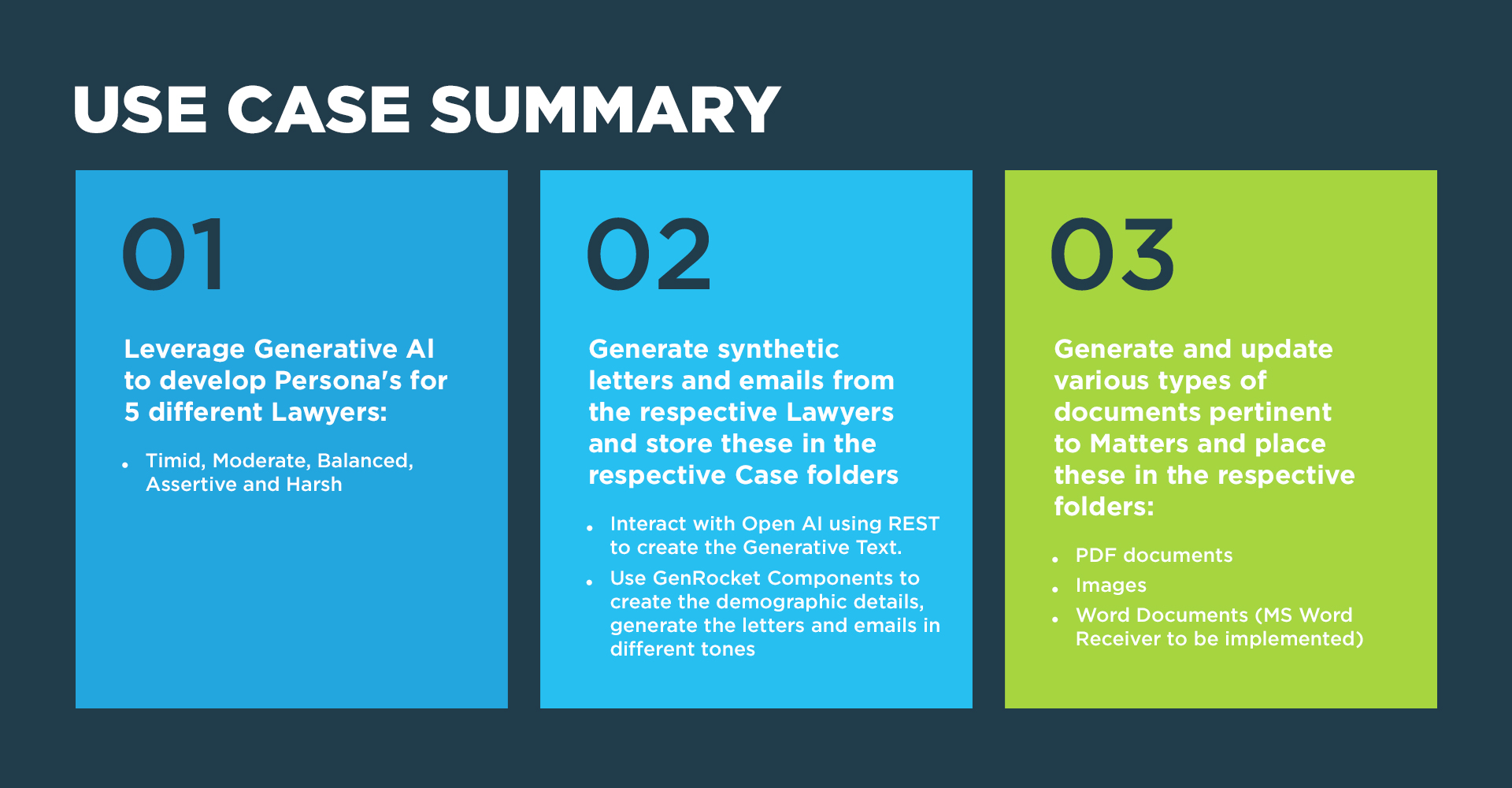
-
Defining the Personas
The legal firm utilized GenRocket, integrated with OpenAI, to create five unique family law personas. These personas ranged from timid to harsh in order to generate their official correspondence with participants in legal disputes and court cases. The personas were defined by prompts used to drive the data generation process within Open AI. By defining these personas and combing the textual correspondence with controlled and structured synthetic data values generated by GenRocket, the platform ensured the generated synthetic data was both relevant and diverse, reflecting a wide range of potential real-world scenarios.
-
Generating Content
Once the GenAI prompts were defined, the next step involved generating the content. OpenAI was utilized to produce letters, emails and other forms of legal documentation. GenRocket’s platform seamlessly integrated the GenAI process with its own capabilities, ensuring the content was enriched with controlled and accurate synthetic data to provide data values such as contact information, case numbers, damages and settlement amounts. This integration allowed for the creation of highly realistic and contextually appropriate documents that could be used for various testing and training purposes.
-
Data Synthesis
The solution went beyond data generation. The legal firm wanted to use GenRocket to combine the controlled tabular data with the AI-generated content and format the data into various family law documents such as divorce petitions, child custody agreements, alimony or child support orders, and other court pleadings. This synthesis of structured and unstructured data showcased the platform’s versatility and its ability to handle complex datasets seamlessly. The ability to merge different types of data is crucial for creating comprehensive test environments that accurately reflect real-world conditions.
-
Document Generation
The firm demonstrated how GenRocket could create different document types, such as PDFs, by synthesizing data and formatting it into any desired document template. This step was crucial in demonstrating how the platform could cater to various data requirements, from generating simple emails to complex, highly formatted legal documents. The flexibility to generate multiple document formats is essential for meeting the diverse needs of the Family Law team as well as other legal departments within the organization.
The Benefits of Integrating GenAI with GenRocket
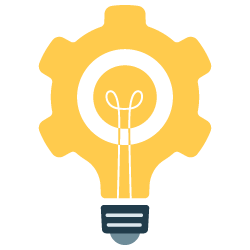
Enhanced Data Quality
By leveraging GenAI for generating textual content and GenRocket for structured data, the platform ensures high-quality and highly customized blended synthetic data values. This combination reduces security risks and improves overall data integrity, providing more reliable data sets for training, testing and development.
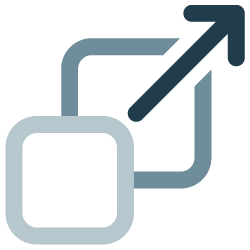
Scalability
GenRocket’s platform is designed to scale, making it suitable for large enterprises with extensive data needs. This use case highlighted how the platform could manage large volumes of data and support multiple users simultaneously, ensuring efficient data provisioning across a wide range of applications. This scalability is vital for organizations that need to rapidly generate and manage large amounts of complex training and test data.
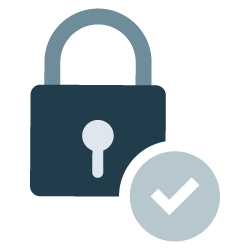
Security
Security is paramount when dealing with synthetic data, especially in regulated industries like law and finance. GenRocket’s secure platform architecture ensures that sensitive data remains protected, offering peace of mind to users. The platform’s robust security measures help organizations comply with strict global data privacy regulations..
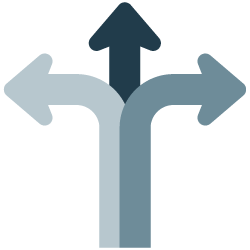
Flexibility
The use case showcased the platform’s flexibility in generating various document types and handling multiple data formats. This flexibility is crucial for enterprises that need to cater to diverse data requirements across different departments. Whether generating simple datasets or complex legal documents, GenRocket adapts to meet specific needs.
Real-World Impact
By integrating GenAI, GenRocket has further enhanced its industry-leading data generation capabilities, making it an invaluable tool for enterprises looking to leverage synthetic data for testing, training, and compliance purposes.
For instance, legal firms can use GenRocket to generate realistic scenarios for training or testing new AI-assisted software applications. Financial institutions can create synthetic datasets to test fraud detection systems without risking sensitive customer information. Healthcare organizations can generate patient data for research and development while ensuring compliance with data privacy regulations. In each of these cases, the ability to produce high-quality synthetic data quickly and securely enables organizations to innovate and improve their services without compromising on data integrity or security.
Conclusion
The integration of GenAI with GenRocket offers a powerful solution for enterprises seeking to enhance their data provisioning capabilities through the use of generative AI technology. By combining the strengths of GenAI and GenRocket’s advanced synthetic data generation platform, GenRocket provides a comprehensive solution that ensures data quality, scalability, security, and flexibility.
It’s becoming very clear that the future of synthetic data provisioning lies in the seamless integration of advanced technologies like Test Data Automation and the various forms and applications of AI. GenRocket is at the forefront of this innovation, offering a robust solution that meets the complex needs of modern enterprises.
In part 4 of this series, we’ll compare the GenRocket solution with alternative synthetic data generation methods and technologies and point out the strengths and weaknesses of each one.