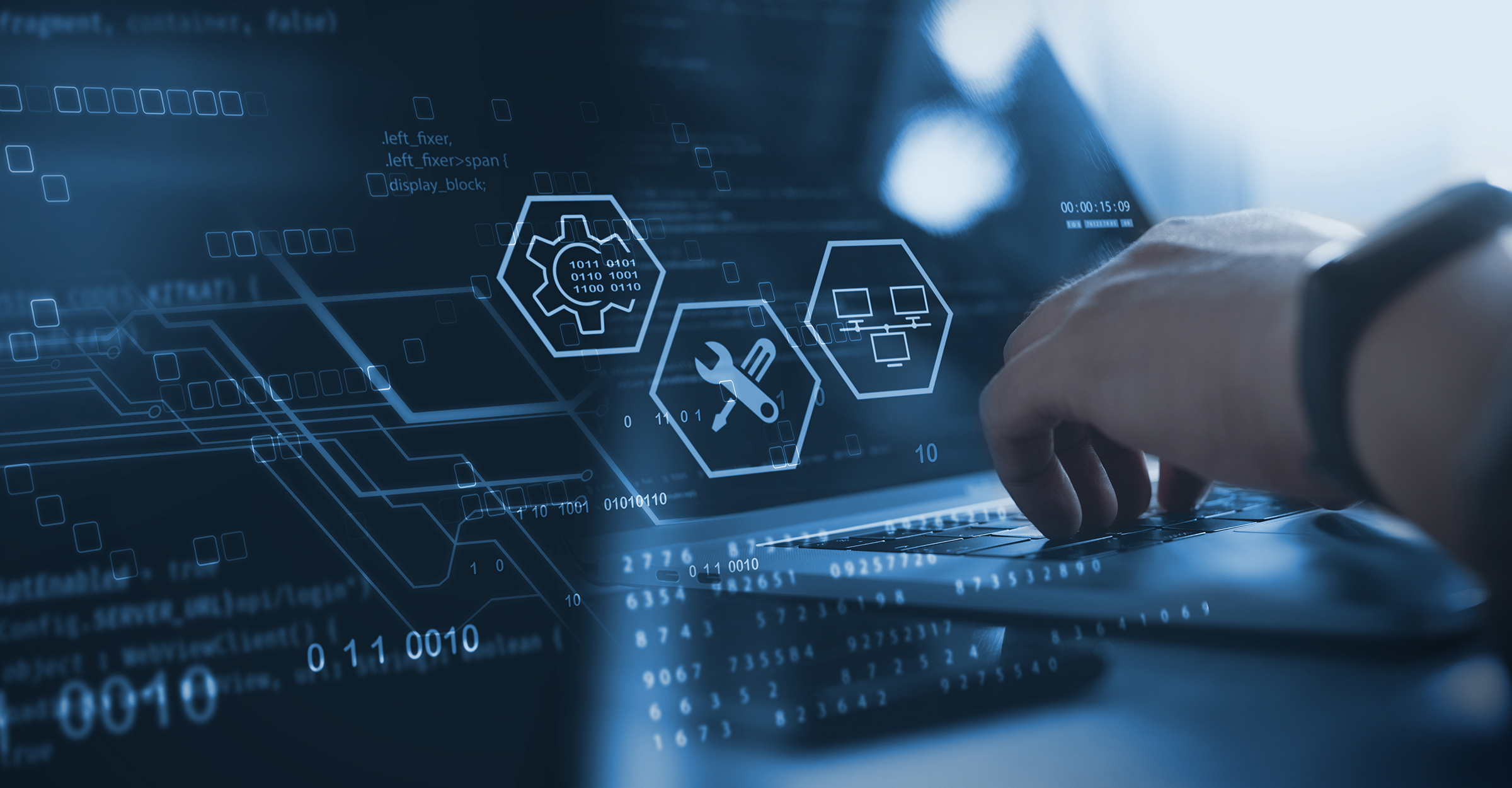
Why Enterprises Are Replacing Production Data with Design-Driven Synthetic Data in Dev and Test Environments
As data privacy regulations tighten and the risk of data exposure grows, enterprises are rethinking their approach to test data management (TDM). In particular, software engineering and quality assurance (QA) teams are facing new pressure from Chief Information Security Officers (CISOs) and compliance officers to stop using masked production data in lower environments. Increasingly, organizations are turning to synthetic data as a secure and scalable alternative. At GenRocket, we’re seeing this shift accelerate across enterprise quality engineering teams with both new and existing customers.
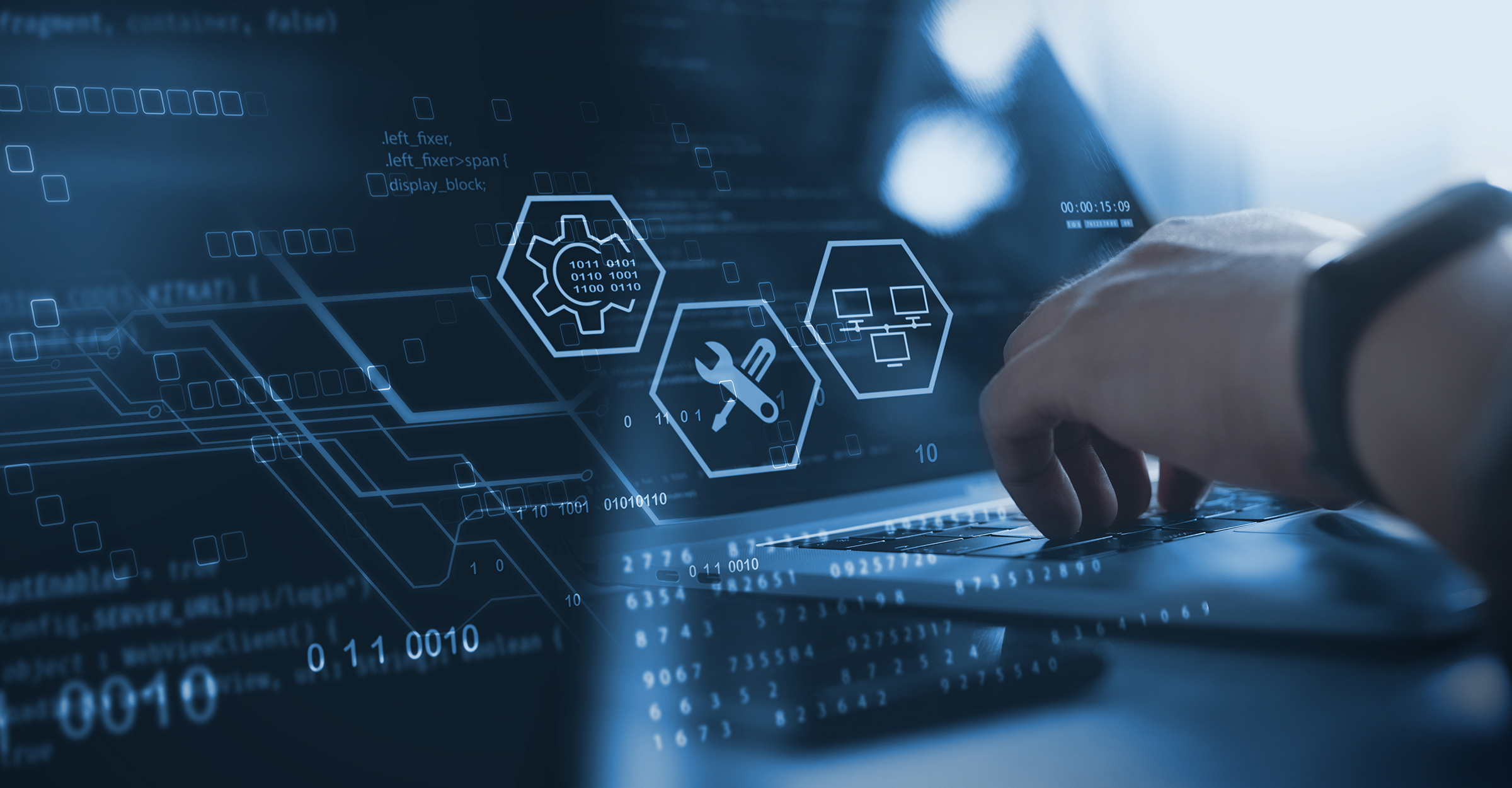
The Privacy Landscape: Stricter Laws, Higher Stakes
With privacy regulations like GDPR, CCPA, HIPAA, and now 20+ U.S. state-specific privacy laws in force, enterprises must now treat data privacy as a board-level concern. A 2024 study by Cisco found that 94% of consumers won’t buy from companies that fail to protect their data.
The stakes are high. IBM’s 2024 Cost of a Data Breach Report found that the average global cost of a data breach rose to $4.88 million, a 10% increase over the previous year. Compounding this, breaches involving development or test environments often go undetected for longer periods and result in more widespread data leakage.
Enterprise Risk and the Shrinking Availability of Production Data
Enterprises are becoming acutely aware of the risks posed by using masked production data in non-production environments. According to a recent Delphix report, 54% of organizations have experienced data breaches in these lower environments, and 86% allow data compliance exceptions in QA, test, and development.
At GenRocket, we’re seeing a growing number of Quality Engineering leaders reporting that their CISOs are blocking access to production data altogether, either now or within the next 12–24 months. This shift stems from a stance that production data should never be exposed, even for a few hours / days during data masking in a lower environment.
This has led organizations to seek TDM platforms that don’t require access to production data at all. GenRocket uniquely meets this requirement by designing and generating synthetic test data based solely on metadata, never needing to store or look at production data.
A Surge in Demand for Synthetic Data
Until recently, synthetic data generation often required explanation to organizations engrained with the traditional TDM paradigm. However, we now routinely engage with organizations asking specifically for synthetic data solutions. This aligns with broader market growth trends. According to Precedence Research, the synthetic data market was valued at $432.08 million in 2024 and is expected to grow at a CAGR of 35.28%, reaching $8.87 billion by 2034.
Key reasons for this growth include:
- Privacy regulations forcing organizations to eliminate sensitive data from dev/test
- Accelerated software delivery cycles requiring fast, reliable test data
- AI/ML adoption that demands high-quality, varied training datasets
Organizations are also realizing that synthetic data can offer much more than compliance. With GenRocket’s unique Design-Driven approach, synthetic data can provide better control, reproducibility, and scalability—allowing teams to generate the exact data they need, when they need it, on-demand and in real-time.
The Need for Hybrid TDM Solutions
While the momentum is shifting toward synthetic data, many enterprises still rely on traditional TDM functionality for specific workflows. For instance, file masking, data subsetting, and production-to-test data migrations still play a role in regulated environments as they begin the transition to synthetic data.
That’s why GenRocket continues to enhance its “one data platform” approach for combining subsets of masked production data with design-driven synthetic data generation. This hybrid model is extremely valuable as organizations gradually phase out direct production data usage in favor of designed synthetic data generation.
The Limits of GenAI for Test Data
Generative AI (GenAI) is another topic frequently raised in conversations with QA leaders. While there’s interest and experimentation underway, experienced teams are already recognizing GenAI’s limitations for test data.
GenAI works well for simple, functional test scenarios, such as generating realistic-looking user profiles or short strings of text. However, it does not scale well to complex enterprise use cases that require referential integrity, deterministic values, and data consistency across systems.
For example, generating synthetic insurance claims that conform to multi-tiered business logic across multiple systems, or healthcare test data with HIPAA-compliant constraints, requires deep structural controls that GenAI currently lacks.
That’s why most enterprise software teams are now concluding that GenAI is a complement, not a replacement for purpose-built synthetic test data platforms. GenRocket has the ability to combine the appropriate use of GenAI for generating textual and conversational datasets along with controlled and conditioned tabular synthetic data created by GenRocket’s massive library of intelligent data generators. This provides our customers with the best of both worlds.
Market Momentum and Strategic Imperatives
As a result, the shift from masked production data to synthetic test data now reflects more than a privacy trend—it’s a strategic transformation in enterprise quality engineering.
Gartner has forecasted that by 2026, 60% of data used in AI and analytics projects will be synthetically generated. The same drivers are now reaching QA and software engineering, where synthetic data helps achieve both data minimization principles under privacy law and speed-to-test objectives under agile and DevOps models.
Furthermore, synthetic data aligns with the zero trust model, which is being widely adopted across enterprise IT. Under zero trust, data should not be assumed safe based on its location or role-based access. Removing sensitive data altogether from testing environments is the most effective way to enforce this model.
Conclusion: Future-Proofing Quality Engineering
Enterprises are at a pivotal moment. Regulatory pressures, CISO policies, and customer expectations are converging to make the use of production data in non-production environments increasingly untenable.
The response isn’t just about compliance—it’s about transformation. Synthetic data offers a path forward that is scalable, efficient, and secure. Synthetic data has moved beyond pilot use into strategic planning and widescale deployment. Organizations are building next-generation QA practices around synthetic data and a clear understanding of where GenAI fits.
As organizations look to future-proof their quality engineering environments, replacing production data with synthetic data will no longer be the exception. It will be the expectation.